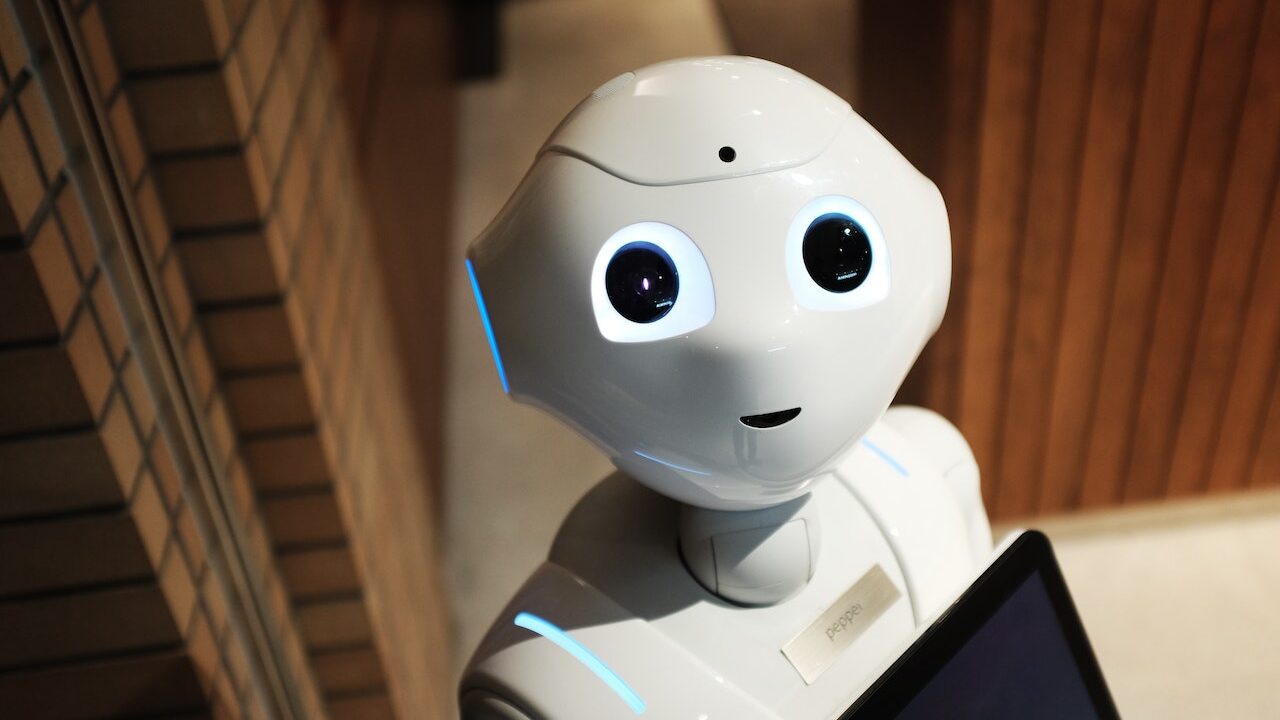
Developing methods and models that allow computers to learn from data and make predictions or judgments without being explicitly programmed is the focus of the artificial intelligence subfield of machine learning. Machine learning is essentially a technique for teaching robots to learn from experience and adapt, much like people do. It entails the application of statistical and mathematical approaches to data analysis, pattern recognition, and prediction or decision-making. Applications for machine learning are numerous, ranging from speech and picture recognition to predictive analytics and autonomous cars. Machine learning is becoming more and more crucial for organizations to get insights and make data-driven decisions as the amount of data generated by businesses and people rises. Machine learning is a topic that is continually changing as new methods and algorithms are created to handle problems that are getting more and more complicated.
Democratization of ML
The practice of making machine learning (ML) more approachable and available to a larger audience is known as “democratizing ML.” In the past, ML was frequently viewed as a specialized area requiring in-depth expertise in computer science, statistics, and mathematics. However, recent developments in ML technology have simplified the use of ML tools and approaches by non-experts to solve issues and get insights from data. The emergence of user-friendly ML platforms, open-source libraries, and cloud-based services that enable people to access and apply ML algorithms without requiring in-depth technical knowledge has sparked this trend toward democratization.
Numerous advantages, such as more innovation, better decision-making, and increased efficiency, may result from the democratization of ML. Businesses, governments, and individuals can learn from data and create novel solutions to challenging issues by making ML more approachable. Healthcare providers can use ML to enhance patient outcomes and cut expenses, while small businesses can use it to analyze consumer data and create tailored marketing strategies.
Machine learning (ML) field in recent years that may continue to influence the field in the coming years.
Deep Learning: Deep learning is a subfield of ML that involves the use of neural networks with many layers, which enables the processing of complex data sets. Deep learning has been used in a wide range of applications, from image and speech recognition to natural language processing.
Reinforcement Learning: Reinforcement learning is a type of ML that involves training agents to make decisions in complex environments by providing feedback in the form of rewards or punishments. This approach has been used in the development of autonomous systems such as self-driving cars and robotics.
Explainable AI: As ML algorithms become more complex, there is a growing need for interpretability and transparency in their decision-making processes. Explainable AI (XAI) is a field that focuses on developing algorithms that can provide explanations for their decisions and enable humans to understand the reasoning behind them.
Federated Learning: Federated learning is a technique that enables ML models to be trained on data sets that are distributed across multiple devices or locations, without the need to transfer data to a central location. This approach can help to address privacy concerns and reduce the computational burden of training large ML models.
Edge Computing: Edge computing involves processing data and running ML algorithms on devices at the edge of a network, such as smartphones and IoT devices, rather than sending data to a central server for processing. This approach can improve performance, reduce latency, and address privacy concerns.
Final Words
Trends and advances in machine learning (ML) in the year 2023. However, current tendencies and developments in the field suggest that ML’s growth and use across industries and applications will continue. Examples include a greater emphasis on explainable AI to ensure interpretability and transparency in decision-making processes, the continuous development and refining of deep learning models for complicated data analysis, and the use of reinforcement learning for autonomous systems.
Further development of federated learning for dispersed data sets and edge computing for data processing on devices at the network’s periphery is also possible. As machine learning develops further, it will be crucial to prioritize the ethical and responsible application of these tools and to guarantee that the advantages of these developments are shared fairly among all members of society.